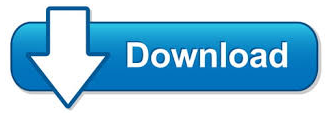
However, in the more advanced statistics and econometrics classes, I learned how to conduct complex regressions in Stata. The ggplot2 package allows you to make high-quality graphs in R in just a few lines, like this graph of average temperature by day that I used in my internship I also find it easier to produce aesthetically pleasing graphs or documents in R as opposed to creating them in Stata. As you might have guessed from the title of the post, I prefer R over Stata: I find it logically easier to understand as it is syntactically quite similar to other coding languages like Java. While the core functionalities are the same, the difference between the two software lies in the way you interact with them and in the way they approach their tasks. You might wonder what is so different between R and Stata. As you begin to search for tools in R that can help you with your analysis, I hope you will find this information useful. This post is a primer of some of the core packages in R that are used for advanced statistical analysis. While our coursework (rightly!) emphasizes the statistical methods, we, as students, are often left to navigate the intricacies of the statistical tools on our own. Usually, students end up developing a preference for one or the other even if they eventually grow proficient in both. Similarly, other departments (for example, for the Undergraduate Certificate Program in Statistics and Machine Learning) offer SML 201 ( Introduction to Data Science) or ORF 245 ( Fundamentals of Engineering Statistics) to prepare students in the use of R. For example, in the Economics program at Princeton, Stata is often the software of choice for classes like ECO 202 ( Statistics and Data Analysis for Economics) or ECO 302/312 (Econometrics). Students who are interested in research – especially junior- and senior-year students preparing for independent work – are often encouraged to master the use of a fully-featured statistical software like Stata or R in order to help with their statistical analysis.
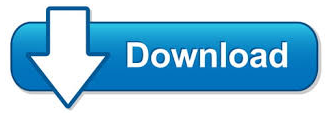